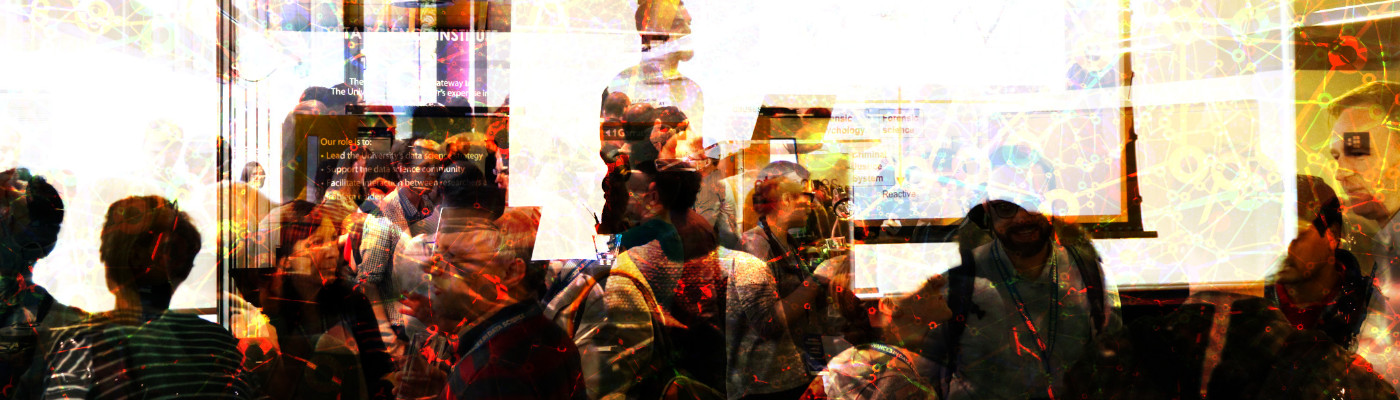
ADSAI 2022 Invited Speakers
Speaker biographies, talk titles and abstracts for all of our invited speakers can be found in the list below.
Virginia Aglietti
Virginia Aglietti is a Research Scientist at Deepmind within the Data Efficient and Bayesian Learning Team. Virginia’s research focuses on causal inference with an emphasis on methods that combine causality and machine learning algorithms for decision making. Prior to joining DeepMind Virginia received a PhD in statistics from the University of Warwick where she was part of the OxWaSP program and she was working on Gaussian processes methods for structured inference and sequential decision making under the supervision of Professor Theo Damoulas.
Virginia’s talk is titled ‘Causal decision-making in static and dynamic settings’
In this talk I will consider the problem of understanding how to intervene in a causal system so as to optimize an outcome of interest. I will present two methodologies that, by linking causal inference, experimental design, and Gaussian process modeling, allow one to efficiently learn the causal effects and identify an optimal intervention to perform, both in static and dynamic settings. In this first part of the talk I’ll focus on static settings and discuss how finding an optimal intervention to perform requires solving a new optimization problem which we call Causal Global Optimization. I’ll then introduce Causal Bayesian Optimization, an algorithm that allows solving these problems by incorporating the knowledge of the causal graph in Bayesian Optimization thus decreasing the optimization cost and avoiding suboptimal solutions. In the second part of the talk, I will then show how the approach developed for static settings can be extended to select actions at different time steps. I’ll discuss how, by successfully integrating observational and interventional information at different time steps, Dynamic Causal Bayesian Optimization allows selecting actions when the causal graph changes over time and the functional relationships among variables differ across time steps. I will conclude by showing the performance of CBO and DCBO on various synthetic and semi-synthetic experiments while discussing their benefits and limitations.
Nikolaos Aletras
Nikolaos Aletras is a Senior Lecturer (~Associate Professor) in Natural Language Processing at the Computer Science Department of the University of Sheffield, co-affiliated with the Machine Learning (ML) group. Previously, he was a Lecturer in Data Science at the Information School, University of Sheffield. Nikolaos has gained industrial experience working as a scientist at Amazon (Amazon ML and Alexa) and was a research associate at UCL, Department of Computer Science. Nikolaos completed his PhD in Natural Language Processing at the University of Sheffield, Department of Computer Science.
Nikolaos’ research interests are in NLP, Machine Learning and Data Science. Specifically, He is interested in developing computational methods for social media analysis and the law, ML for NLP and information retrieval methods for improving access to large document collections. His research has been funded by the EPSRC, ESRC, Leverhulme Trust, EU, Amazon and Google.
Nikolaos’ conference talk is titled: ‘How can we improve explanation faithfulness in NLP’.
Large pre-trained language models (LLMs) currently dominate performance across language understanding tasks. However, their complexity and opaque nature have opened up new challenges on how to extract faithful explanations (or rationales), which accurately represent the true reasons behind a model’s prediction when adapted to downstream tasks. In this talk, I will present recent work from my group on how we can improve faithfulness of LLM predictions and a study of explanation faithfulness in out-of-domain settings.
Umang Bhatt
Umang Bhatt is a Ph.D. candidate in the Machine Learning Group at the University of Cambridge. His research lies in trustworthy machine learning. Specifically, he focuses on algorithmic transparency and its effects on stakeholder decision-making. His work has been supported by a JP Morgan AI PhD Fellowship, a Mozilla Fellowship, and a Partnership on AI Research Fellowship. He is currently an Enrichment Student at The Alan Turing Institute, a Student Fellow at the Leverhulme Centre for the Future of Intelligence, and an Advisor to the Responsible AI Institute. Previously, he was a Fellow at the Mozilla Foundation and a Research Fellow at the Partnership on AI. Umang received a B.S. and M.S. in Electrical and Computer Engineering from Carnegie Mellon University.
Umang’s talk is titled ‘Challenges and Frontiers in Deploying Transparent Machine Learning’
Explainable machine learning offers the potential to provide stakeholders with insights into model behavior, yet there is little understanding of how organizations use these methods in practice. In this talk, we will discuss recent research exploring how organizations view and use explainability. We find that most deployments are not for end-users but rather for machine learning engineers, who use explainability to debug the model. There is thus a gap between explainability in practice and the goal of external transparency since explanations are primarily serving internal stakeholders. Providing useful external explanations requires careful consideration of the needs of stakeholders, including end-users, regulators, and domain experts. Despite this need, little work has been done to facilitate inter-stakeholder conversation around explainable machine learning. To help address this gap, we report findings from a closed-door, day-long workshop between academics, industry experts, legal scholars, and policymakers to develop a shared language around explainability and to understand the current shortcomings of and potential solutions for deploying explainable machine learning in the service of external transparency goals.
Sylvie Delacroix
Professor Delacroix’s research focuses on the intersection between law and ethics, with a particular interest in habits and the infrastructure that moulds our habits (data-reliant tools are an increasingly big part of that infrastructure).
She is considering the potential inherent in bottom-up Data Trusts as a way of reversing the current top-down, fire-brigade approach to data governance. She co-chairs the Data Trust Initiative, which is funded by the McGovern Foundation: see https://datatrusts.uk.
Professor Delacroix has served on the Public Policy Commission on the use of algorithms in the justice system (Law Society of England and Wales) and the Data Trusts Policy group (under the auspices of the UK AI Council). She is also a Fellow of the Alan Turing Institute. Professor Delacroix’s work has been funded by the Wellcome Trust, the NHS and the Leverhulme Trust, from whom she received the Leverhulme Prize. Her latest book -Habitual Ethics?- is forthcoming with Bloomsbury / Hart Publishing in July 2022. @SylvieDelacroix | https://delacroix.uk
Professor Delacroix’s conference presentation, is titled ‘Data trusts as bottom-up data empowerment infrastructure’.
It proceeds from an analysis of the particular type of vulnerability concomitant with our ‘leaking’ data on a daily basis, to argue that data ownership is both unlikely and inadequate as an answer to the problems at stake.
There are three key problems that bottom-up data trusts seek to address:
- Lack of mechanisms to empower groups, not just individuals
- Can we do better than current ‘make belief’ consent?
- Can we challenge the assumed tradeoff between promoting data-reliant common goods on one hand and addressing vulnerabilities that stem from data sharing?
Melanie Fernandez Pradier
Melanie is a senior researcher at Microsoft Research working on probabilistic models for healthcare and the live sciences. Her research interests include generative models for Immunomics, Bayesian modelling, interpretable ML, and out-of-distribution generalization. Melanie received her PhD on Bayesian Nonparametrics from University Carlos III in 2017. She was a postdoctoral fellow at the Harvard Data Science Initiative until 2020, working on interpretable ML and deep Bayesian models with Finale Doshi-Velez. Melanie is a co-founder of the ICBINB Initiative, and an editor of the MDPI special issue on “Foundations and Challenges of Interpretable ML”.
Melanie’s talk is titled ‘From the research to the clinic: aligning ML systems with clinicians for improved mental care’
Current Machine Learning (ML) approaches can achieve high levels of performance on clinical data that matches or even exceeds human clinicians, e.g., by leveraging the power of deep neural networks and large data repositories. However, success stories of deploying ML systems in healthcare domains remain limited. Lack of interpretability and misalignment with clinical expertise are some frequent caveats of such systems. In this talk, I will share the insights that my colleagues and I learned when trying to bridge the gap from the research to the clinic in the context of antidepressant prescriptions. I will start with a word of caution based on a within-subject factorial user study: ML recommendations and explanations may impact clinicians decisions negatively. I will then present preferential MoE, a novel human-ML mixture-of-experts model that rely on human expertise as much as possible, demonstrating its potential in the management of Major Depressive Disorder.
Darminder Ghataura
Dr. Darminder Ghataoura is Head of AI and leads Fujitsu’s offerings and capabilities in AI and Data Science within the Defence and National Security space, acting as Technical Design Authority with responsibility for shaping proposals and development of integrated AI solutions.
Darminder manages the strategic and technical AI relationships with partners, academic institutions and UK government and is a Strategic Advisory Network member for the UKRI Trustworthy Autonomous Systems (TAS) Hub, advising on its research direction and identifying impact opportunities to accelerate the adoption of Trusted AI solutions developed within the Programme.
Darminder is also a Simon Industrial fellow at the University of Manchester , Decision and Cognitive Sciences Department , with his main focus in the area of ‘Human machine-teaming’.
Darminder has over 15 years’ experience in the design and development of AI systems and services across the UK Public and Defence sectors as well as UK and international commercial businesses.
He was awarded with the Fujitsu Distinguished Engineer recognition in 2020 and holds an Engineering Doctorate (EngD) in Autonomous Military Sensor Networks for Surveillance Applications, from University College London (UCL).
Darminder’s conference talk is titled ‘Open challenges for closer Human-Machine Teaming’
Interactions with artificial intelligence (AI) is now commonplace. We increasingly rely on intelligent systems to extend our human capabilities, from chat-bots that provide technical support to virtual assistants like Siri and Alexa. However, today’s intelligent machines are essentially tools, not true collaborative partners.
If the vision for Human-Machine teaming is to augment human processes and improve productivity, intelligent machines will need to be flexible and adaptive to the states of the human, as well as the environment. This poses interesting challenges such as understanding human capabilities, intentions, the ability of the machine to generalise to new situations and the all important trust dimension. In this talk we will look to highlight some of these challenges and to understand where we are currently on this journey.
David Leslie
David Leslie is the Director of Ethics and Responsible Innovation Research at The Alan Turing Institute. Before joining the Turing, he taught at Princeton’s University Center for Human Values, where he also participated in the UCHV’s 2017-2018 research collaboration with Princeton’s Center for Information Technology Policy on “Technology Ethics, Political Philosophy and Human Values: Ethical Dilemmas in AI Governance.” Prior to teaching at Princeton, David held academic appointments at Yale’s programme in Ethics, Politics and Economics and at Harvard’s Committee on Degrees in Social Studies, where he received over a dozen teaching awards including the 2014 Stanley Hoffman Prize for Teaching Excellence. He was also a 2017-2018 Mellon-Sawyer Fellow in Technology and the Humanities at Boston University and a 2018-2019 Fellow at MIT’s Dalai Lama Center for Ethics and Transformative Values.
David has served as an elected member of the Bureau of the Council of Europe’s Ad Hoc Committee on Artificial Intelligence (CAHAI). He is on the editorial board of the Harvard Data Science Review (HDSR) and is a founding editor of the Springer journal, AI and Ethics. He is the author of the UK Government’s official guidance on the responsible design and implementation of AI systems in the public sector, Understanding artificial intelligence ethics and safety (2019) and a principal co-author of Explaining decisions made with AI (2020), a co-badged guidance on AI explainability published by the Information Commissioner’s Office and The Alan Turing Institute. He is also Principal Investigator of a UKRI-funded project called PATH-AI: Mapping an Intercultural Path to Privacy, Agency and Trust in Human-AI Ecosystems, which is a research collaboration with RIKEN, one of Japan’s National Research and Development Institutes founded in 1917. Most recently, he has received a series of grants from the Global Partnership on AI, the Engineering and Physical Sciences Research Council, and BEIS to lead a project titled, Advancing Data Justice Research and Practice, which explores how current discourses around the problem of data justice, and digital rights more generally, can be extended from the predominance of Western-centred and Global North standpoints to non-Western and intercultural perspectives alive to issues of structural inequality, coloniality, and discriminatory legacies.
David was a Principal Investigator and lead co-author of the NESTA-funded Ethics review of machine learning in children’s social care (2020). His other recent publications include the HDSR articles “Tackling COVID-19 through responsible AI innovation: Five steps in the right direction” (2020) and “The arc of the data scientific universe” (2021) as well as Understanding bias in facial recognition technologies (2020), an explainer published to support a BBC investigative journalism piece that won the 2021 Royal Statistical Society Award for Excellence in Investigative Journalism. David is also a co-author of Mind the gap: how to fill the equality and AI accountability gap in an automated world (2020), the Final Report of the Institute for the Future of Work’s Equality Task Force and lead author of “Does AI stand for augmenting inequality in the COVID-19 era of healthcare” (2021) published in the British Medical Journal. He is additionally the lead author of Artificial intelligence, human rights, democracy, and the rule of law (2021), a primer prepared to support the CAHAI’s Feasibility Study and translated into Dutch and French, and of Human rights, democracy, and the rule of law assurance framework for AI systems: A proposal. In his shorter writings, David has explored subjects such as the life and work of Alan Turing, the Ofqual fiasco, the history of facial recognition systems and the conceptual foundations of AI for popular outlets from the BBC to Nature.
David’s talk is titled ‘From principles to practice and back again: Building a responsible AI ecosystem from the ground up’
The recent history of AI ethics and governance has been characterised by increasingly vocal calls for a shift from principles to practice. In this talk, I will explore some of shortcomings of those who have championed this shift. I will argue that many staunch supporters of the “principles to practice” movement have, in fact, taken too much of a documentation- and audit-centred point of view, thereby neglecting the social, cultural, and cognitive preconditions of the responsible innovation practices they aspire to advance. Beyond off-the-shelf tools and documentation-centred governance instruments, closing the gap between principles and practice requires a transformation of organisational cultures, technical approaches, and individual attitudes from inside the processes and practices of design, development, and deployment themselves. Achieving this requires researchers, technologists, and innovators to establish and maintain end-to-end habits of critical reflection and deliberation across every stage of a research or innovation project’s lifecycle.
Christina Lioma
Christina is Professor in Computer Science at the University of Copenhagen.
Christina Lioma is a professor in machine learning at the Department of Computer Science, University of Copenhagen. Her research focuses on applied machine learning, information retrieval and web search technologies, web data mining and analytics, recommendation systems and natural language processing. She has a track-record of research collaboration with Danish and international industry, and an alumni of >20 PhD students and postdocs. Since 2012, Christina Lioma has attracted more than 50 million USD in external funding.
Christina’s talk is titled ‘Pitfalls with ablation in neural network architectures’
Abstract:
Ablation tests are frequently used for analysing machine learning performance. Generally, ablation refers to the removal of a component in order to understand its contribution to the overall decision-making process. For instance, it is expected that the ablation of a feature during classification will affect the output somehow analogously to the importance of that feature for the classification task at hand. Ablation is therefore routinely used to attribute feature importance, as well as to explain machine learning output in a partial, approximate, and model-agnostic way.
This talk will point out a core problem when using ablation with neural network architectures. The problem stems from the tendency of neural network architectures to ignore complex predictive features in the presence of few simple predictive features, even when the complex features have significantly greater predictive power than the simple features. This talk will provide evidence demonstrating the existence of this tendency, even in small neural network architectures, and show how this may entirely invalidate the standard interpretation of ablation tests. A discussion about why this is important and ways of moving forward will be provided.
This is joint work with Qiuchi Li (University of Copenhagen).
André Martins
André Martins (PhD 2012, Carnegie Mellon University and University of Lisbon) is an Associate Professor at Instituto Superior Técnico, University of Lisbon, researcher at Instituto de Telecomunicações, and the VP of AI Research at Unbabel. His research, funded by a ERC Starting Grant (DeepSPIN) and other grants (P2020 project Unbabel4EU and CMU-Portugal project MAIA) include machine translation, quality estimation, structure and interpretability in deep learning systems for NLP. His work has received best paper awards at ACL 2009 (long paper) and ACL 2019 (system demonstration paper). He co-founded and co-organizes the Lisbon Machine Learning School (LxMLS), and he is a Fellow of the ELLIS society.
André’s talk is titled ‘Towards Explainable and Uncertainty-Aware NLP’
Abstract:
Natural language processing systems are becoming increasingly more accurate and powerful. However, in order to take full advantage of these advances, new capabilities are necessary for humans to understand model predictions and when to question or to bypass them. In this talk, I will present recent work from our group in two directions.
In the first part, I will describe how sparse modeling techniques can be extended and adapted for facilitating sparse communication in neural models. The building block is a family of sparse transformations called alpha-entmax, a drop-in replacement for softmax, which contains sparsemax as a particular case. Entmax transformations are differentiable and (unlike softmax) they can return sparse probability distributions, useful for explainability. Structured variants of these sparse transformations, SparseMAP and LP-SparseMAP, are able to handle constrained factor graphs as differentiable layers, and they have been applied with success to obtain deterministic and structured rationalizers, with favorable properties in terms of predictive power, quality of the explanations, and model variability, with respect to previous approaches.
In the second part, I will present an uncertainty-aware approach to machine translation evaluation. Recent neural-based metrics for translation quality such as COMET or BLEURT resort to point estimates, which provide limited information at segment level and can be unreliable due to noisy, biased, and scarce human judgements. We combine the COMET framework with two uncertainty estimation methods, Monte Carlo dropout and deep ensembles, to obtain quality scores along with confidence intervals. We experiment with varying numbers of references and further discuss the usefulness of uncertainty-aware quality estimation (without references) to flag possibly critical translation mistakes.
This is joint work with Taya Glushkova, Nuno Guerreiro, Vlad Niculae, Ben Peters, Marcos Treviso, and Chryssa Zerva in the scope of the DeepSPIN and MAIA projects.
Naoaki Okazaki
Naoaki Okazaki is Professor in School of Computing, Tokyo Institute of Technology, Japan.
Prior to this faculty position, he worked as a post-doctoral researcher at the University of Tokyo (in 2007-2011), and as an associate professor at Tohoku University (2011-2017). He is also a visiting research scholar of the Artificial Intelligence Research Center (AIRC), National Institute of Advanced Industrial Science and Technology (AIST). His research areas include Natural Language Processing (NLP), Artificial Intelligence (AI), and Machine Learning.
Naoaki’s talk is titled ‘Towards controllable, faithful, and explainable text generation’
Abstract:
Deep neural networks have made a breakthrough in various NLP tasks in the recent ten years. Benchmark scores of neural machine translation were nearly doubled from those of the statistical machine translation with the advancements in sequence-to-sequence models, attention mechanism, and the Transformer architecture. This success also broadened natural language generation (NLG) applications into abstractive summarization and image caption generation. However, relying on a large amount of supervision data, the current NLG models are not flexible to adapt to a slightly different task, reduce unreliable outputs, and explain the reason behind outputs. In this talk, I will present our recent studies on controllable and faithful abstractive summarization and explainable grammatical error correction.
Alfredo Vellido
Alfredo Vellido is Associate Professor in Computer Science at the Polytechnic University of Catalonia.
Alfredo completed a B.Sc. in Physics at the Universidad del País Vasco (UPV-EHU), Spain in 1996. He earned his Ph.D in Neural Computation at Liverpool John Moores University (LJMU). Alfredo then became Ramón y Cajal Research Fellow at Universitat Politécnica de Catalunya (UPC), Barcelona, Spain (2003-2008) and has been Associate Professor at UPC since then.
His research focuses on ML applications in healthcare and medicine and, lately, on their societal impact.
Alfredo’s talk is titled ‘Explain yourself: XAI as social responsibility’.
Machine-learning-based systems are now part of a wide array of real-world applications seamlessly embedded in the social realm. In the wake of this realization, strict legal regulations for these systems are currently being developed, addressing some of the risks they may pose. This is the coming of age of the concepts of interpretability and explainability in machine-learning-based data analysis, which can no longer be seen just as an academic research problem.