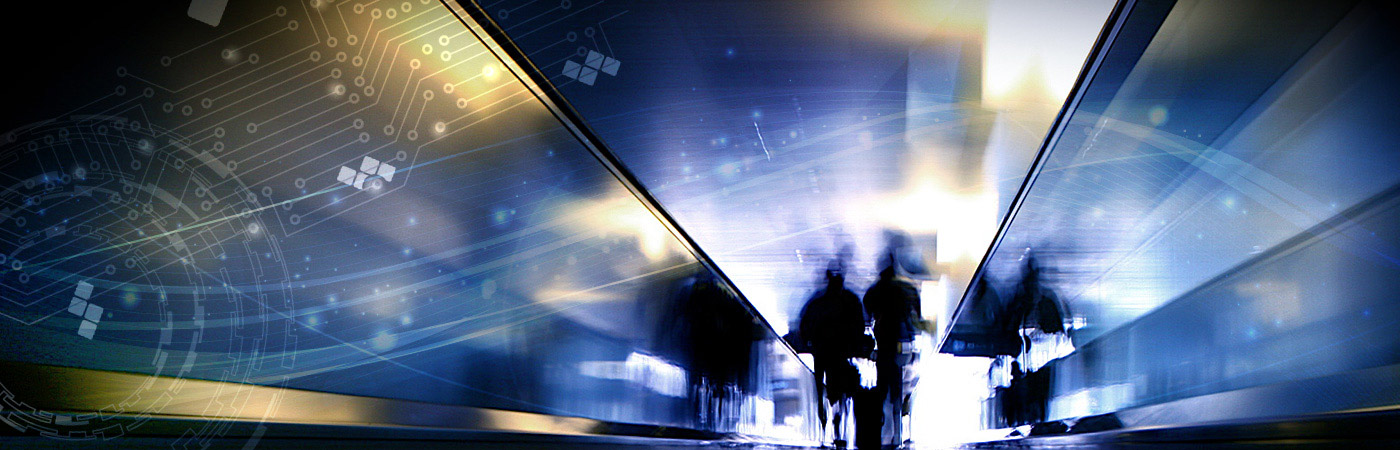
Alessandra Parisio
Reader in the Department of Electrical and Electronic Engineering
What are your research interests?
My main research interests include the areas of energy management under uncertainty, model predictive control, stochastic constrained control, distributed optimisation for power systems. In particular, my research focuses on the design of advanced control and optimisation frameworks to manage flexibility (i.e., the ability to adjust to the time-varying network conditions) in urban districts and in energy networks as a whole. Flexibility plays a crucial role for the transition towards power systems that can efficiently accommodate high shares of renewable energy sources. There are multiple sources of flexibility (e.g., storage devices, electric vehicles, demand technologies), and several multi-energy systems within a district (i.e., systems with interconnected electricity/heating/gas networks), which currently lack coordination and can be regarded as excellent flexibility providers. Novel control and optimisation strategies, and transformative decision-making schemes, are needed to harness their unique potential.
What is the focus of your current research?
The current focus is mainly on the analysing the role and the use of digital technologies and data to assist in handling complex decisions and improving control and management of flexibility across multiple interconnected energy networks. Data-based methods, probabilistic and physics-informed machine learning, set-based techniques (e.g., deep neural networks, Gaussian processes, ambiguity sets) can inform and enhance energy network solutions. Advanced data-driven control algorithms need to be developed to enable more scalable and efficient decision making, leveraging data and algorithms from different ICT devices and locations, through a more informed optimal management of interconnected multi-energy flexible resources, increased energy efficiency, mitigated network imbalances and asset degradation. We are currently exploring the use of data to derive control-oriented dynamic models of the system and of the environment, to automatically learn the control inputs, to obtain automatic tuning of the cost parameters and constraints, to incorporate feedforward law into the control design (e.g., Optimisation-based ramping reserve allocation of BESS for AGC enhancement, IEEE Transactions on Power Systems, 2023).
What are some projects or breakthroughs you wish to highlight?
With £5 million funding from the EPSRC, the Supergen Energy Networks Impact Hub will investigate the modernisation of energy transmission and distribution systems to make them a driving force towards a rapid, safe, and just transition to net zero. Within this project, one work package, which I am leading, is focused on “Digitalisation”, which aims to demonstrate the transformational potential of digitalisation to help create a highly interconnected and flexible multi-energy network.
What memberships and awards do you hold/have you held in the past?
- IEEE PES Outstanding Engineer Award in January 2021
- Energy and Buildings Best Paper Award for (for 2008-2017) in January 2019
- Co-Chair of the Technical Committee of the IEEE Robotic and Automation Society on Smart Buildings, since November 2021
- Vice-Chair for Education of the Technical Committee 9.3 of IFAC on Control for Smart Cities, since December 2020
- Member of the Evaluation Panel for the Swiss National Science Foundation (SNSF) Starting Grants since January 2022
- Member of the European Control Association Conference Editorial Board (EUCA-CEB), since 2022
- IEEE Senior Member since October 2018
What is the biggest challenge in Data Science and AI right now?
There are several challenges in Data Science, considering in particular a control perspective; they include no definite theory, need for large amounts of data, lack of robustness and vulnerability to adversarial attacks, lack of transparency and explainability, need to systematically characterise the uncertainty, computational reproducibility.
What real world challenges do you see Data Science and AI meeting in the next 25 years?
Besides the challenges mentioned above, one of the main real-world challenges is the development of an architecture that enables data to be accessible and shared across multiple energy sectors, hence facilitating the deployment of the digital tools. This challenge is closely linked to the challenges of interoperability, standardised data sharing and cyber-security.